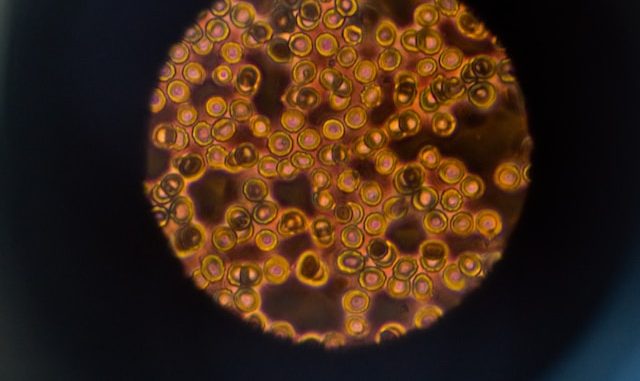
Spatial transcriptomics (ST) isn’t just a buzzword in the field of molecular biology; it’s a monumental leap, bridging our knowledge of gene expression with their intricate locations within tissues. Just as a map is meaningless without coordinates, understanding gene expression without its spatial context was a limiting factor in molecular biology. The traditional practice of grinding up tissue for data has evolved, and now we have the tools to see the bigger, more detailed picture.
Reflecting its significance, the global spatial genomics and transcriptomics market was valued at an impressive $232.5 million in 2022. Moreover, this market is not just holding steady; it’s projected to flourish, with an anticipated CAGR of 12.2% from 2023 to 2030. This growth trajectory underlines the transformative potential and the widespread interest in ST across biomedical research sectors.
Basics of Spatial Transcriptomics
The beauty of spatial transcriptomics lies in its approach. Traditionally, when we wanted to know what genes were active, we’d grind up a piece of tissue and sequence everything, losing all spatial information. ST, on the other hand, keeps that spatial information intact.
This technique allows researchers to visualize gene expression patterns directly in tissue sections, preserving the intricate architecture of biological structures. The essence of ST is to correlate ‘gene expression’ with ‘location’, giving a new depth to our understanding.
Techniques & Methodologies
Delving deeper into the science, the first step in ST is tissue preparation. This ensures that the tissue maintains its structural integrity during the process. Once the tissue is prepared, high-resolution imaging techniques are employed to capture a detailed picture of the tissue morphology.
The crux of spatial transcriptomics is the capturing of transcript data. Using specially designed arrays or sequencing methodologies, each mRNA molecule is tagged with a unique spatial barcode that corresponds to its position in the tissue. This ensures that, post-sequencing, every bit of transcriptomic data can be mapped back to its exact location in the tissue section.
Data Analysis in Spatial Transcriptomics
With great data comes great responsibility. The rich datasets generated from ST are both a boon and a challenge. Bioinformatics plays a pivotal role in making sense of this data. Typically, specialized software and pipelines are employed to process, analyze, and visualize the transcriptomic data in its spatial context.
But it’s not without hurdles. The intricacies of aligning sequence data with spatial information, ensuring accuracy, and interpreting spatial patterns are tasks that require both powerful computational resources and innovative analytical strategies.
Applications in Biomedical Research
The applications of spatial transcriptomics in biomedical research are vast and transformational. One primary application is the study of tissue architecture and organization. By observing how genes are expressed in a spatial context, researchers can gain insights into how tissues develop, function, and degenerate.
Moreover, ST offers a window into the world of cellular interactions. Cells aren’t solitary; they communicate, interact, and function as part of a complex network. By understanding the spatial aspect of gene expression, we can better comprehend these cellular dialogues, especially in microenvironments, such as tumor niches.
Diseases, too, have a spatial aspect. The progression of many diseases can be traced as a cascade of molecular events across tissue space. ST, with its ability to map these events, promises groundbreaking insights into pathology and, consequently, has vast potential in the realms of drug discovery and personalized medicine.
Limitations and Challenges
However, like any pioneering technique, spatial transcriptomics, though transformative, comes with its share of inherent challenges. Technically speaking, the resolution, depth, and sensitivity of current methods may not capture every single transcript, especially those expressed at extremely low levels or in dense regions. The sheer complexity, volume, and size of the data generated require advanced, sophisticated storage solutions and infrastructures.
Furthermore, interpretation, despite sophisticated tools, isn’t always straightforward. Ensuring unbiased readings, considering cellular diversity, and making sense of intricate spatial patterns demand both acute caution and profound expertise.
Future Perspectives
The horizon of spatial transcriptomics is radiant with possibilities. Emerging technologies promise to enhance resolution and depth, capturing an even more detailed spatial transcriptomic landscape.
Additionally, the integration of ST with other ‘omics’ techniques, like proteomics and genomics, can weave a holistic picture of cellular function and regulation. This synthesis of technologies, in turn, holds significant promise for both clinical and translational research, paving the way for innovative treatments and therapeutic strategies.